Gone are the days of manual, repetitive testing and endless spreadsheets. As software development takes center stage, AI in testing is advancing to revolutionize quality assurance. Due to smarter algorithms detecting flaws, predictive failures, and automating tests, organizations today are able to achieve faster, more accurate results.
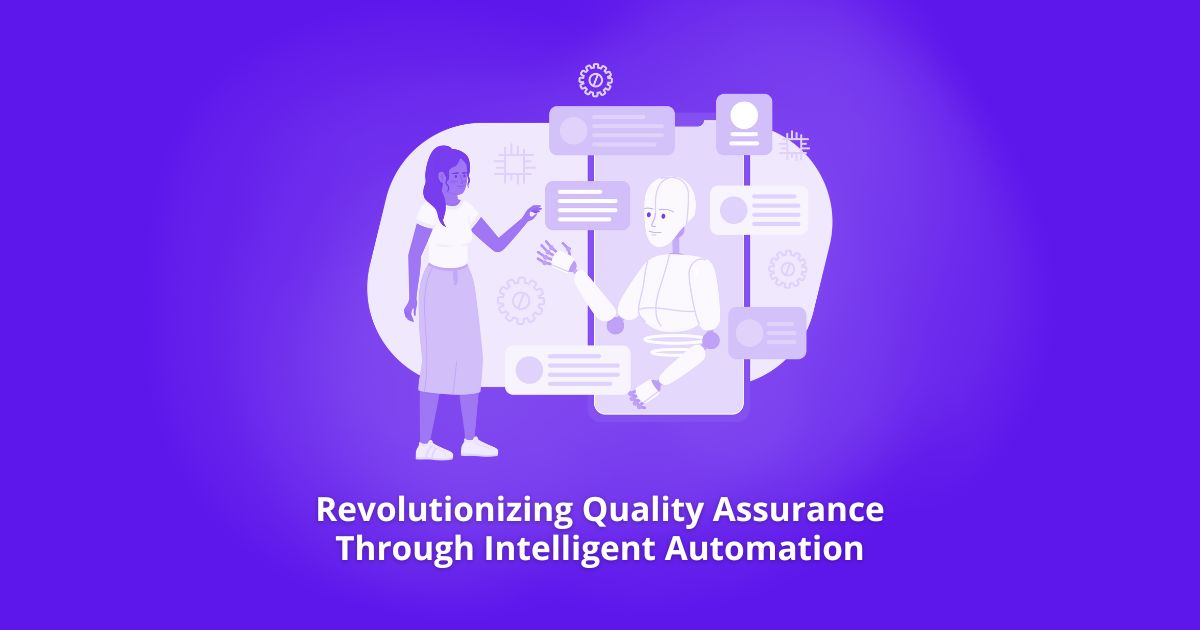
Imagine a future where AI does the mundane work, freeing testers to spend time developing an extraordinary user experience. It’s not just efficiency – it’s intelligent automation transforming QA. In this blog, we’ll explore how AI is reshaping testing, the role of tools like LambdaTest, and why integrating AI into your strategy is a game-changer.
The Game-Changing Role of AI in Testing
There was a time when testing consisted of tedious checking, spreadsheets ad infinitum, and many a coffee-fuelled, tension-filled late night – not a little like driving in a jam during rush hour with your GPS laughing at your expense. Thankfully, such times are slipping into the rearview mirror. With AI in testing, organizations are finding new avenues for simplifying their QA processes, reducing human errors, and overall enhancing product quality.
Imagine a future in which tedious, repetitive work is handled by smart machines. AI algorithms can now scan through codes, detect faults, and even predict future failure locations even when actual faults have not yet developed. It’s a kind of crystal ball through which technology groups can glimpse into the future and correct faults even when they have not yet made an unsightly presence. Not only is it a timesaver, but it helps testers work towards what actually matters: enhancing user experiences.
The Power of Analytics
AI is revolutionizing software testing by providing actionable insights based on user behavior, app performance, and environmental variables. This data-driven approach helps teams make informed decisions, refine their testing strategies, and significantly enhance software quality.
To harness AI effectively, you need the right tools—and LambdaTest stands out as a leader in this space. As an AI-powered test orchestration and execution platform, LambdaTest enables teams to run manual and automated tests at scale across 5,000+ real devices, browsers, and OS combinations.
What sets LambdaTest apart is its seamless AI integration. Its GenAI-powered agent, Kane AI, helps optimize test execution, detect anomalies, and enhance efficiency. By leveraging intelligent automation, teams can ensure cross-device compatibility while reducing manual effort—allowing testers to focus on strategy rather than repetitive tasks.
Testing AI: A New Frontier
As companies embrace testing AI, it’s no longer just about running automated tests. It’s about using AI’s potential to enhance the testing process. This bold new frontier involves integrating machine learning techniques, enabling systems to learn from past outcomes and improve over time. Picture an app that becomes progressively smarter, learning from user interactions to catch bugs before they even happen. That’s the future we are heading toward.
Think of AI testing like a superhero with a sidekick. The sidekick (us, the humans) establishes the mission parameters, but the Artificial intelligence brings the tremendous power and speed to see it through. Through robust algorithms and predictive analytics, teams can enhance their testing strategies, making them more effective and less time-consuming.
Real-World Applications: Virtual Success Stories
But is this just wishful thinking? Not at all! Companies embracing AI in testing are already seeing substantial benefits. For instance, consider a major online retail company. By implementing AI-driven testing tools, they handled large volumes of transactions during holiday sales without a hitch. The organization used AI to predict traffic spikes and adjust their test cases accordingly, ensuring the site remained responsive even during peak times. As a result, they not only maintained customer satisfaction but also saw increased sales year over year.
Another example is a software development app that transitioned from testing through a conventional, manual process to AI-enabled automation. By automating its regression tests, it freed its testing assets in excess of 60%, and engineers could utilize them for developing new feature development and not bug fixing. With such a transition, its time-to-market reduced significantly, and overall productivity saw an improvement. Now, they can roll out updates every few weeks instead of monthly, keeping them ahead of the competition.
The Human Element in Automated Testing
While AI in software testing is instrumental in transforming QA, let’s not forget the importance of the human touch. The aim isn’t to replace testers but to empower them. Engagement and innovation come when people collaborate with technology. Testers bring contextual awareness that AI might not fully comprehend. They understand customer concerns and user experience nuances in ways an algorithm might overlook.
Consider a scenario where an AI system can automatically run hundreds of test cases. However, it doesn’t take into account the empathy behind a user’s frustration when navigating a confusing interface. Humans are required to speak on behalf of the user – the very reason why understanding the user journey remains crucial in a data-driven world.
Integrating AI with Human Efforts
To find the sweet spot between human insight and AI capabilities, a collaborative approach should be taken. Teams can use AI for repetitive testing work and free experienced, creative testers for testing user experiences and exploratory testing. Organizations can drive productivity and produce innovation with a blended model, developing a rich and memorable user experience that will make them stand out in a competitive marketplace.
Challenges in AI-Driven Testing
Of course, with a rose comes a thorn, and with AI providing such a wealth of value to QA, comes its own range of specific obstacles, too. For one, there’s the issue of bias and data integrity. AI algorithms draw a lot of value from training data that’s entered into them. In the event that training data is not complete, and/or is biased, tests generated through them can yield incorrect information. AI tools must receive uncorrupted, unbiased data in a position to generate effective output. Ensure, therefore, that whatever information you’re entering it with is uniform, correct, and as representative in character as can possibly be.
There’s also a danger of over-dependency on automation. Organizations can fall into a trap of thinking that AI will simplify all of their work, and human supervision can go out of the window. Don’t forget, even the best AI algorithms require guidance and fine-tuning a little, remember? It’s worth having experienced testers who can interpret AI feedback, help re-adjust testing strategies, and ensure that user experience doesn’t become secondary in terms of value in the pecking order.
Best Practices for Implementing AI in Testing
Integrating AI does not just mean automating processes – it means maximizing efficiency, accuracy, and flexibility while still keeping human expertise central. In achieving the best out of AI-enabled testing,
consider these best practices below:
➜ Start with Clear Objectives
Before implementing AI, define what you want to achieve. Are you looking to reduce test execution time, improve defect detection, or enhance test coverage? Aligning AI capabilities with your QA goals ensures meaningful results.
➜ Use AI for Repetitive and Data-Intensive Tasks
AI shines when handling large datasets and automating repetitive tests. Utilize AI for regression testing, visual testing, and performance monitoring while allowing human testers to focus on exploratory testing and user experience.
➜ Train AI with Quality Data
AI algorithms rely on data for intelligent decision-making. Make your training datasets diverse, unbiased, and representative of real-world situations to avoid incorrect test outcomes and improve predictive analytics.
➜ Combine AI with Human Expertise
While AI can optimize test execution, it lacks the intuition and domain knowledge of human testers. Use AI as a powerful assistant rather than a replacement, ensuring that testers provide oversight, validate results, and fine-tune testing strategies.
➜ Leverage AI for Self-Healing Tests
Test scripts often break due to UI or functional changes. AI-driven self-healing capabilities automatically update test cases when applications evolve, reducing maintenance efforts and ensuring seamless test execution.
➜ Monitor and Continuously Improve AI Models
AI-driven testing is not a set-it-and-forget-it solution. Regularly monitor AI performance, fine-tune models, and analyze test results to improve accuracy and adapt to new challenges.
➜ Ensure Security and Compliance
When using AI-enabled testing instruments, you need to confirm both their security protocols and industry standards. AI-enabled automation should not compromise data privacy, nor compromise any kind of regulatory conformity.
By adhering to these best practices, companies are able to unlock AI’s full power in testing, achieving increased quality of software, accelerated releases, and an optimal user experience.
Current Trends and Future Outlook
As technology continues to develop, so will testing with AI. On the horizon is one such development: codeless testing platforms, through which testers can build and run tests with no need for them to produce any code. With such democratization of testing, it is becoming increasingly simple for non-development professionals to become a part of testing processes. Imagine the power of a marketing professional being able to validate the functionality of the website they are promoting – all done with the help of intelligent automation!
Additionally, the technology surrounding AI models is rapidly advancing. Natural Language Processing (NLP) technology continues to become smarter, with AI reading and processing language in a manner that’s becoming ever more similar to humans. That could mean one day, it’s simply a matter of explaining what you want to try, and the system will understand and execute it – now that’s ease taken to a whole new level!
Conclusion
In a nutshell, the rise of AI in testing is indeed revolutionizing the QA landscape. It’s enabling teams to work more efficiently, respond to user needs with precision, and enhance product quality. While challenges remain, the integration of intelligent automation and human insights represents the future of quality assurance. So why not embrace the transformations that lie ahead? After all, in the race for quality, the only constant is change – a change worth investing in if you want to stay ahead of the curve.
As technology continues evolving, it’s time for you to pick up the instruments and processes offered by tools like LambdaTest and infuse this intelligent automation into quality assurance plans. By marrying AI capabilities with human capabilities, you are able to drive your processes of testing to uncharted territory, giving users the best possible digital experience. The digital environment changes, so must you.
So, go grab your coffee (or tea, for that matter) and sit down to begin taking your first steps towards a future in which quality assurance redefines its purpose through unmatched capabilities of smart automation and AI. Your users – and your team – will thank you for it!